Track 2: FlatCam for Faces: Enhancement, Reconstruction, and Verification
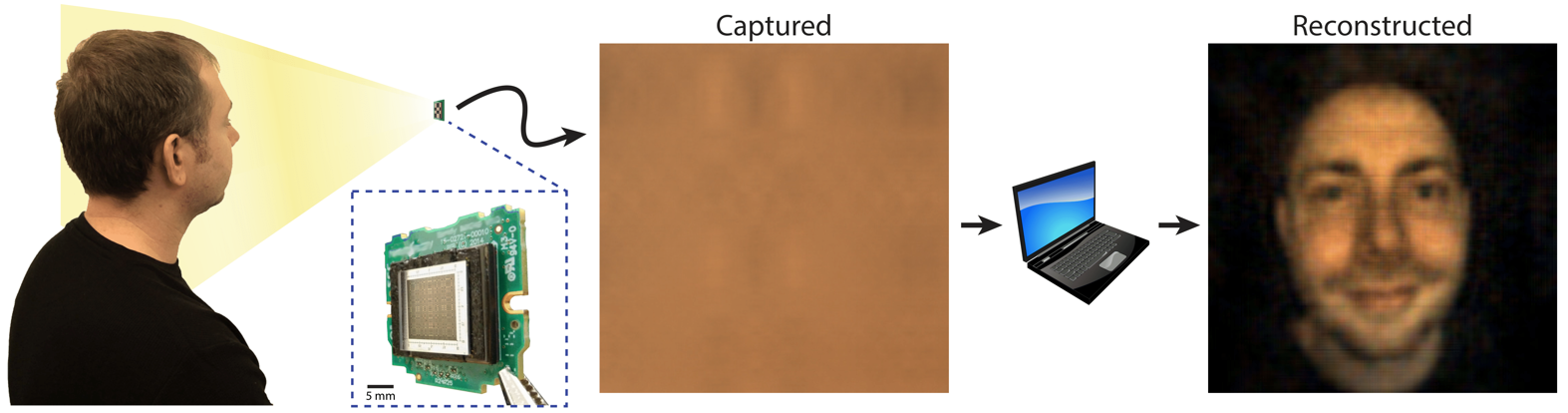
Registration form: LINK
Advances in computer vision and machine learning continuously give rise to an increasing number of applications. However, some of these applications, such as the Internet of Things, lightweight drones, etc., have strict size and cost constraints that standard lens-based cameras (with costs of ≈ $20 and thicknesses of ≈ 7mm) cannot satisfy. The FlatCam is a new lensless camera design from computational photography that aims to address these concerns by having a thickness of ≈ 1mm and a predicted production cost of < $1 at scale. Such a design can be more easily incorporated into highly constrained settings, allowing image inference tasks to be performed in stringent applications.
The FlatCam replaces the optical lenses of a standard lens-based camera with a thin amplitude mask containing multiple apertures and an accompanying image reconstruction algorithm [2]. Due to the absence of optical focusing elements and because of non-idealities in the reconstruction, FlatCam images come with a unique set of artifacts that have yet to be closely studied. What has been shown, however, is that applying a standard off-the-shelf face verification algorithm on FlatCam images without any modification to the algorithm yields very low accuracies even if the same algorithm performs very well on webcam images of the same scenes [1]. Thus, there is still a missing step before one can use the wealth of computer vision algorithms as is on FlatCam images. This step may be filled in by a proper image enhancement algorithm or by a vision-specific reconstruction algorithm, both of which are desired outcomes of this challenge.
In addition, for applications solely interested in performing a recognition task, image reconstruction may not even be necessary. It may be possible to obtain even better recognition performance by designing an algorithm that takes as inputs the unreconstructed FlatCam measurements and outputs the desired predictions without including an intermediate image reconstruction step. Direct recognition on coded computational photography measurements is relatively unstudied, and a third desired outcome of this challenge is an algorithm that can successfully do so on FlatCam images.
UG2+ Challenge 2 aims to improve the accuracy of face verification on FlatCam images via three different approaches represented by the following three sub-challenges:
- Image Enhancement for FlatCam Face Verification
- Image Reconstruction for FlatCam Face Verification
- End-to-End Face Verification on FlatCam Measurements
In all three sub-challenges, the participant teams are allowed to use external training data that are not mentioned above, including self-synthesized or self-collected data; but they must state so in their submissions.
If you have any questions about this challenge track please feel free to email cvpr2020.ug2challenge.track2@gmail.com
References:
[1] J. Tan, L. Niu, J. Adams, V. Boominathan, J. T. Robinson, R. G. Baraniuk, and A. Veeraraghavan, "Face Detection and Verification Using Lensless Cameras," in IEEE Trans. Comput. Imag., vol. 5, no. 2, pp. 180-194, June 2019.
[2] S. M. Asif, A. Ayremlou, A. Sankaranarayanan, A. Veeraraghavan, and R. G. Baraniuk, "FlatCam: Thin, Lensless Cameras Using Coded Aperture and Computation," in IEEE Trans. Comput. Imag., vol. 3, no. 3, pp.384-397, July 2016.
Footer