Track 3: Single Image Deraining
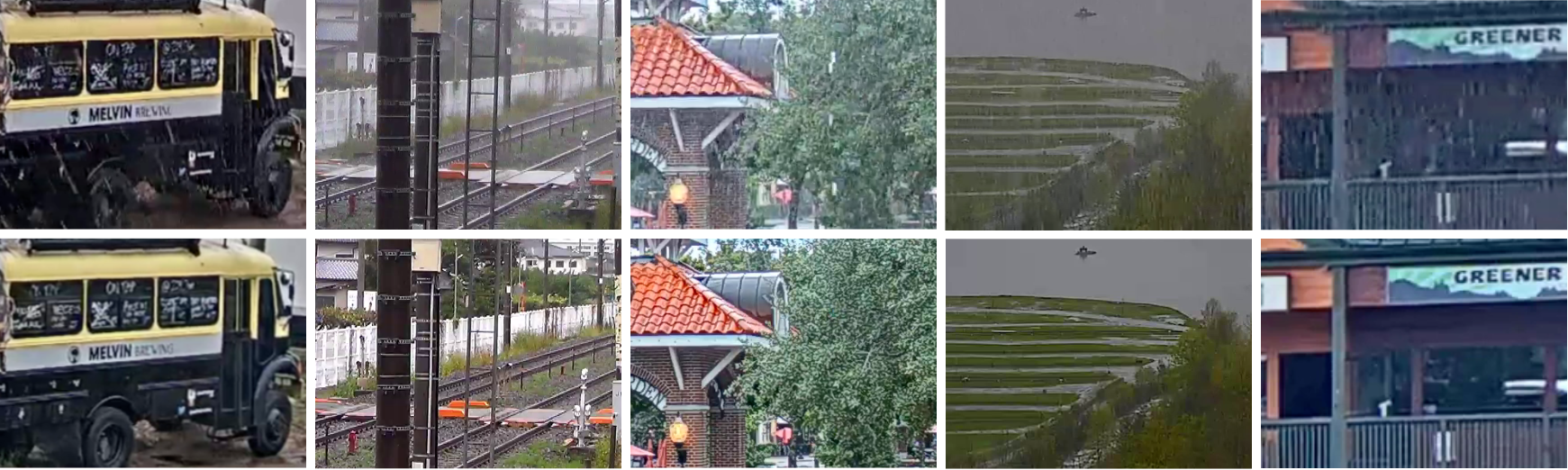
The GT-RAIN challenge invites the public to push the boundary of single image deraining for challenging real world images degraded by various degrees of rainy weather that were collected from all around the world—stretching from North America to Asia. The competition features the first large scale dataset comprised of real rainy image and ground truth image pairs captured from over 115 scenes. The challenge is sponsored by the US Army Research Laboratory (ARL) with monetary awards for the best performing teams: $1000 USD for first place, $800 USD for second place and $500 USD for third place.
Single-image deraining aims to remove degradations induced by rain from images. Top performing methods use deep networks, but suffer from a common issue: it is not possible to obtain ideal real ground-truth pairs of rain and clean images. The same scene, in the same space and time, cannot be observed both with and without rain. To overcome this, deep learning based rain removal relies on synthetic data. However, current rain simulators cannot model all the complex effects of rain which leads to unwanted artifacts when applying models trained on them to real-world rainy scenes. For instance, a number of synthetic methods add rain streaks to clean images to generate the pair, but rain does not only manifest as streaks: If raindrops are further away, the streaks meld together creating rain accumulation, or veiling effects, which are exceedingly difficult to simulate. A further challenge with synthetic data is that results on real test data can only be evaluated qualitatively, for no real paired ground truth exists.
To address these limitations of synthetic data, the GT-RAIN dataset [1] was proposed. Unlike existing datasets, GT-RAIN consists of pairs of real rainy image and ground truth image captured moments after the rain had stopped -- the negative performance impact induced by short temporal interval is much less than that of synthetic data. Paired videos collected within a short time frame of each other are strictly filtered with objective criteria on illumination shifts, camera motions, and motion artifacts. Further correction algorithms are applied for subtle variations, such as slight movements of foliage. GT-RAIN features diverse and challenging scenarios that include (i) various types of rain conditions (i.e. long and short streaks, various densities and accumulation, with and without rain fog), (ii) large variety of background scenes from urban locations (i.e. buildings, streets, cityscapes) to natural scenery (i.e. forests, plains, hills), (iii) varying degrees of illumination from different times of day, and all of which are captured by (iv) cameras that cover a wide array of resolutions, noise levels, and intrinsic parameters.
The aim of this competition is to study the rainy weather phenomenon in real world scenarios and to spark innovative ideas that will further the development of single image deraining methods on real images. In a collaboration with the authors of GT-RAIN, we have further collected an additional 15 extra scenes set aside as the official benchmark competition test set where performance evaluation will computed quantitatively. As a part of the competition, each team must register on the competition website and must use the GT-RAIN dataset to train their models. Teams are open to use additional datasets to push performance, but must include details of them in a README file included in the submission to the competition.
The logistics of Track 3 is handled seperately. For details regarding the datasets, rules and prizes, please check the CodaLab Page of the challenge.
If you have any questions about this challenge track please feel free to email GTRainChallenge@gmail.com
References:
[1] Ba, Y., Zhang, H., Yang, E., Suzuki, A., Pfahnl, A., Chandrappa, C.C., de Melo, C.M., You, S., Soatto, S., Wong, A. and Kadambi, A., 2022. Not Just Streaks: Towards Ground Truth for Single Image Deraining. In European Conference on Computer Vision (pp. 723-740). Springer, Cham.
Footer