The rapid development of computer vision algorithms increasingly allows automatic visual recognition to be incorporated into a suite of emerging applications. Some of these applications have less-than-ideal circumstances such as low-visibility environments, causing image captures to have degradations. In other more extreme applications, such as imagers for flexible wearables, smart clothing sensors, ultra-thin headset cameras, implantable in vivo imaging, and others, standard camera systems cannot even be deployed, requiring new types of imaging devices. Computational photography addresses the concerns above by designing new computational techniques and incorporating them into the image capture and formation pipeline. This raises a set of new questions. For example, what is the current state-of-the-art for image restoration for images captured in non-ideal circumstances? How can inference be performed on novel kinds of computational photography devices?
Continuing the success of the 1st (CVPR'18), 2nd (CVPR'19), 3rd (CVPR'20), 4th (CVPR'21), and 5th (CVPR'22) UG2 Prize Challenge workshops, we provide its 6th version for CVPR 2023. It will inherit the successful benchmark dataset, platform and evaluation tools used by the previous UG2 workshops, but will also look at brand new aspects of the overall problem, significantly augmenting its existing scope.
Original high-quality contributions are solicited on the following topics:
- Novel algorithms for robust object detection, segmentation or recognition on outdoor mobility platforms (UAVs, gliders, autonomous cars, outdoor robots etc.), under real-world adverse conditions and image degradations (haze, rain, snow, hail, dust, underwater, low-illumination, low resolution, etc.)
- Novel models and theories for explaining, quantifying, and optimizing the mutual influence between the low-level computational photography tasks and various high-level computer vision tasks, and for the underlying degradation and recovery processes, of real-world images going through complicated adverse visual conditions.
- Novel evaluation methods and metrics for image restoration and enhancement algorithms, with a particular emphasis on no-reference metrics, since for most real outdoor images with adverse visual conditions it is hard to obtain any clean “ground truth” to compare with.
Challenge Categories
Winners
$K
Awarded in prizes
Keynote speakers
Available Challenges
What is the current state-of-the-art for image restoration for images captured in non-ideal circumstances? How can inference be performed on novel kinds of computational photography devices?
The UG2+ Challenge seeks to advance the analysis of "difficult" imagery by applying image restoration and enhancement algorithms to improve analysis performance. Participants are tasked with developing novel algorithms to improve the analysis of imagery captured under problematic conditions.
Object Detection in Haze
While most current vision systems are designed to perform in environments where the subjects are well observable without (significant) attenuation or alteration, a dependable vision system must reckon with the entire spectrum of complex unconstrained and dynamic degraded outdoor environments. It is highly desirable to study to what extent, and in what sense, such challenging visual conditions can be coped with, for the goal of achieving robust visual sensing.
Our challenge is based on the A2I2-Haze, the first real haze dataset with in-situ smoke measurement aligned to aerial and ground imagery.
Atmospheric Turbulence Mitigation
The theories of turbulence and propagation of light through random media have been studied for the better part of a century. Yet progress for associated image reconstruction algorithms has been slow, as the turbulence mitigation problem has not thoroughly been given the modern treatments of advanced image processing approaches (e.g., deep learning methods) that have positively impacted a wide variety of other imaging domains (e.g., classification).
This challenge aims to promote the development of new image reconstruction algorithms for incoherent imaging through anisoplanatic turbulence.
Single Image Deraining
Images captured in adverse weather conditions significantly impact the performance of many vision tasks. Rain is a common weather phenomenon that introduces visual degradations to captured images and videos through partial occlusions of objects – in heavy rain, severe occlusion to the background. As most vision algorithms assume clear weather, with no interference of rain, their performance suffers. Deraining is the task of removing such visual degradations so that the images are better suited to the assumptions of downstream vision algorithms, as well as for aesthetic fruition.
This challenge aims to spark innovative ideas that will push the envelope of single image deraining on real images.
Keynote speakers
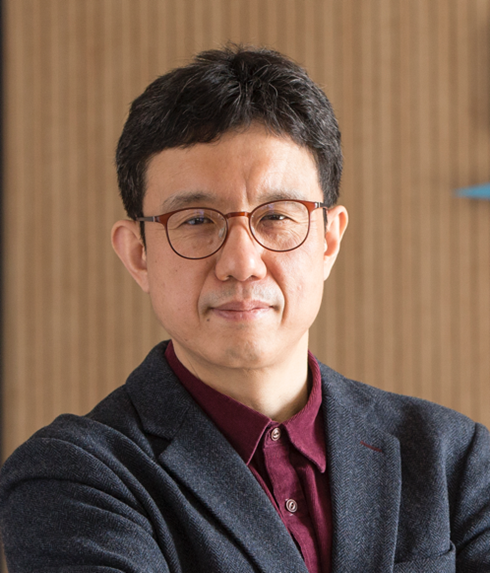
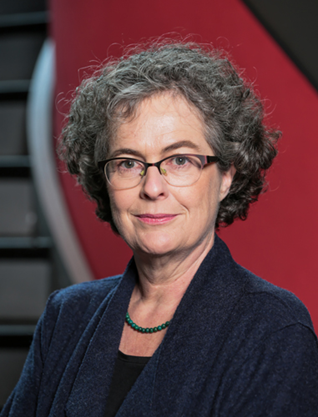
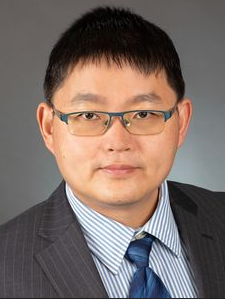
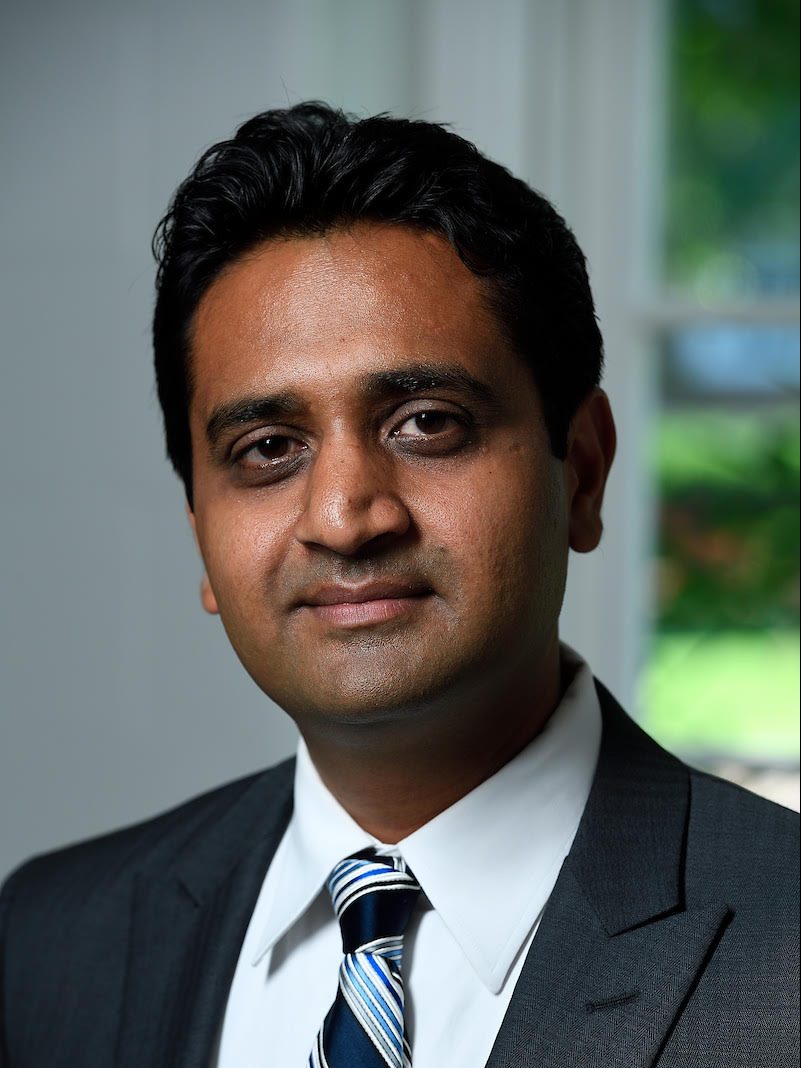
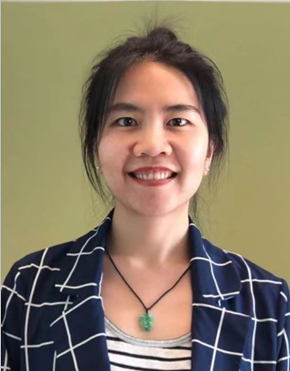
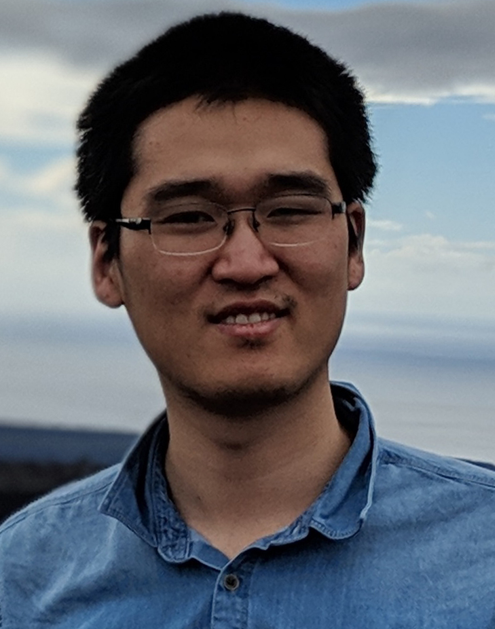
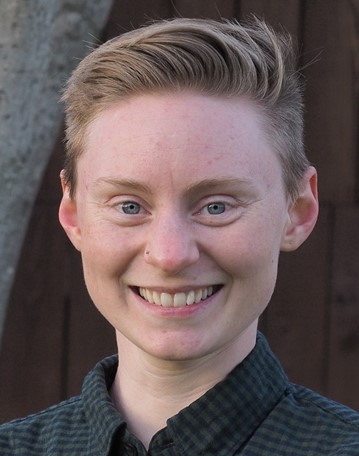
Important Dates
Challenge Registration
January 15 - May 1, 2023
Challenge Dry-run
January 15 - May 1, 2023
Paper Submission Deadline
March 22, 2023
Notification of Paper Acceptance
March 31, 2023
Paper Camera Ready
April 4, 2023
Challenge Final Result Submission
April 30 - May 1, 2023
Challenge Winners Announcement
May 25, 2023
CVPR Workshop
June 19, 2023
Advisory Committee
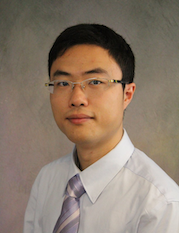
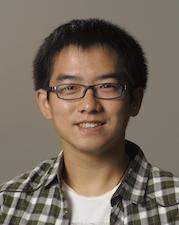
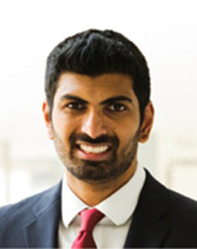
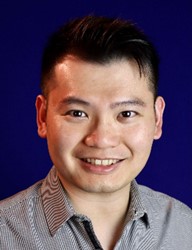
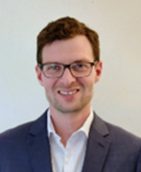
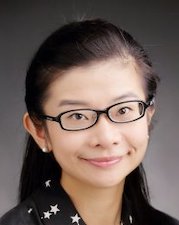
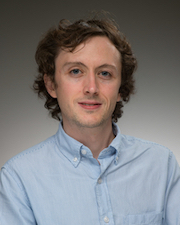
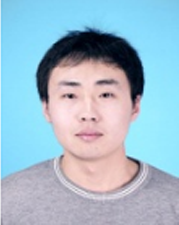
Organizing Committee
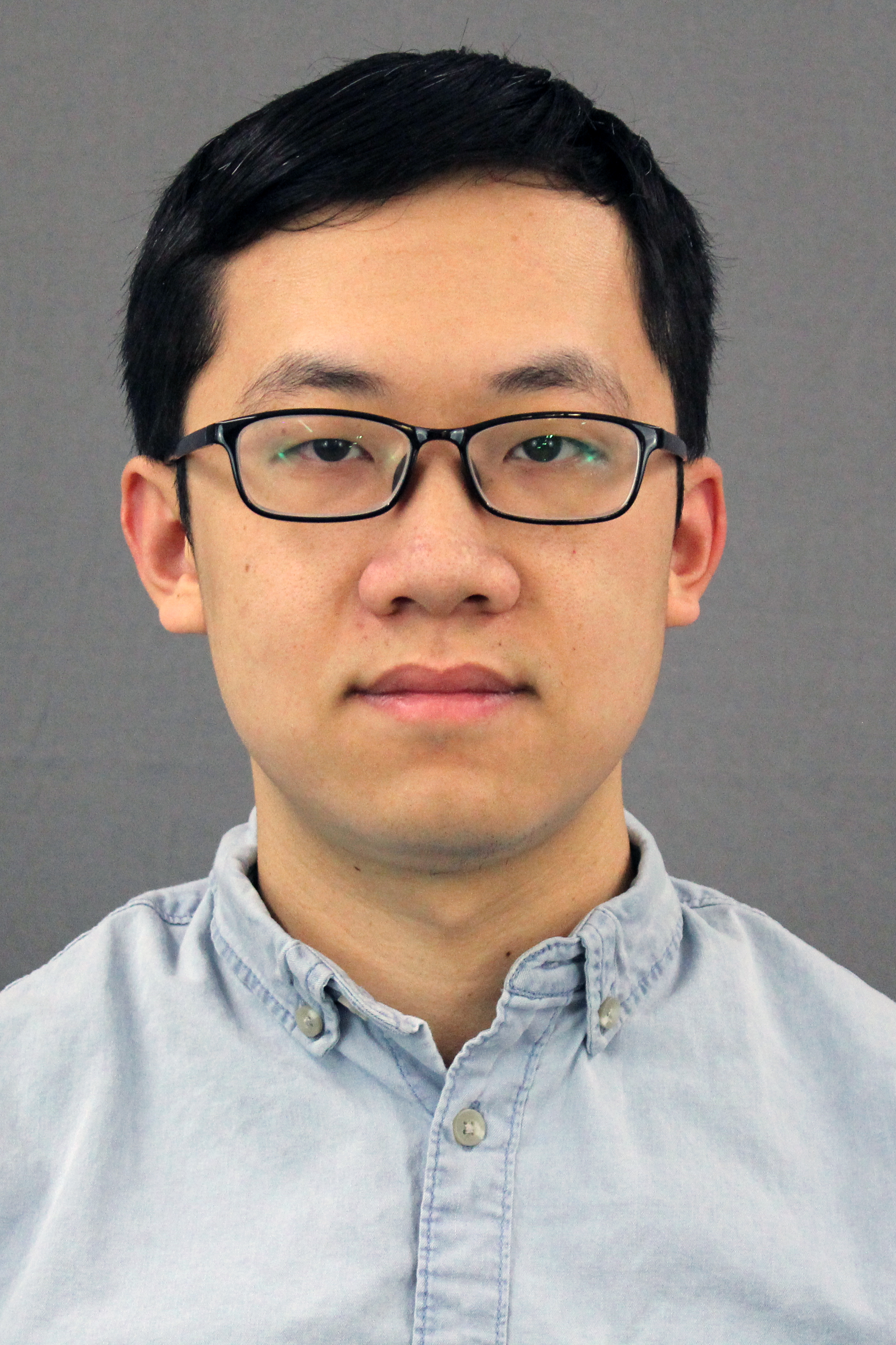
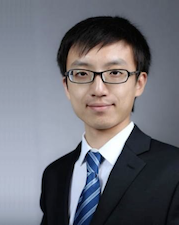
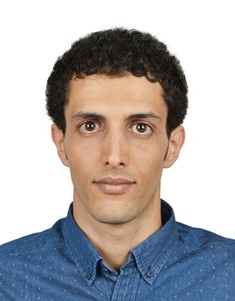
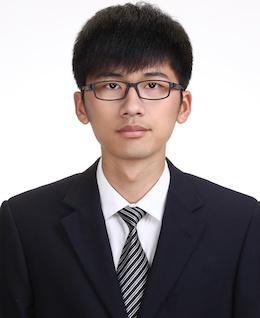
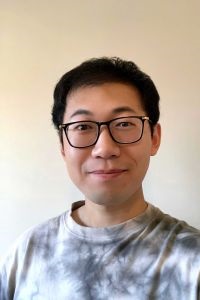
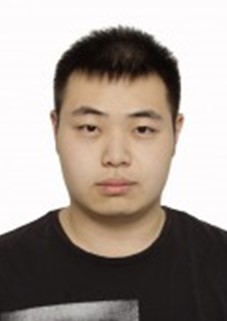
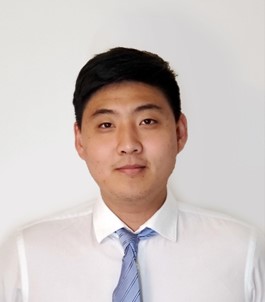